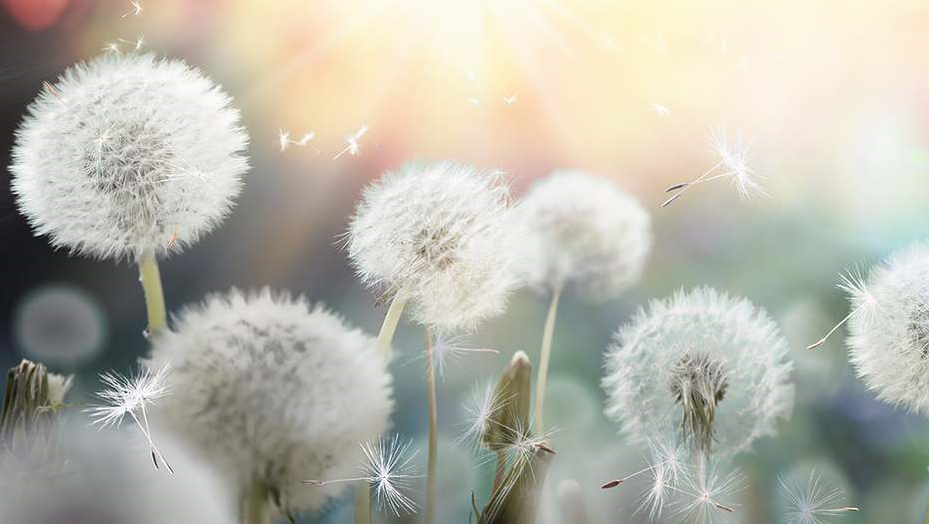
This introductory course will help you to understand what open science is and why it is something you should care about. You'll get to grips with the expectations of research funders and will learn how practising aspects of open science can benefit your career progression. Upon completing this course, you will:
- understand what Open Science means and why you should care about it
- be aware of some of the different ways to go about making your own research more open over the research lifecycle
- understand why funding bodies are in support of Open Science and what their basic requirements are
- be aware of the potential benefits of practicing open science
It is important to remember that Open Science is not different to traditional science. It just means that you carry out your research in a more transparent and collaborative way. Open Science applies to all research disciplines. While Open Science is the most commonly used term, you may also hear people talking about Open Scholarship or Open Research in the Arts and Humanities.
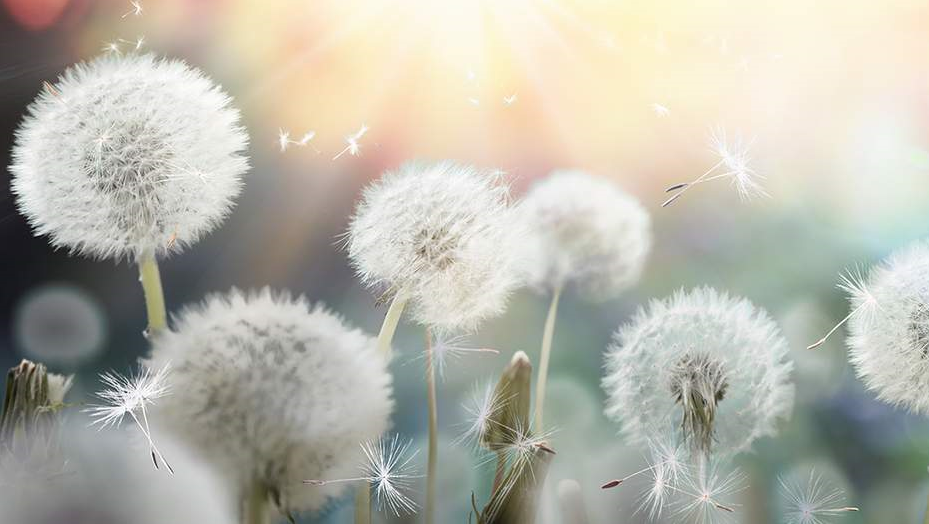
Data-driven research is becoming increasingly common in a wide range of academic disciplines, from Archaeology to Zoology, and spanning Arts and Science subject areas alike.
To support good research, we need to ensure that researchers have access to good data. Upon completing this course, you will:
- understand the differences between open, closed, and shared data,
- be able to make decisions about which data you can share,
- know what a Data Management Plan (DMP) is,
- be aware of the FAIR principles,
- know how to get maximum impact from sharing your research data.
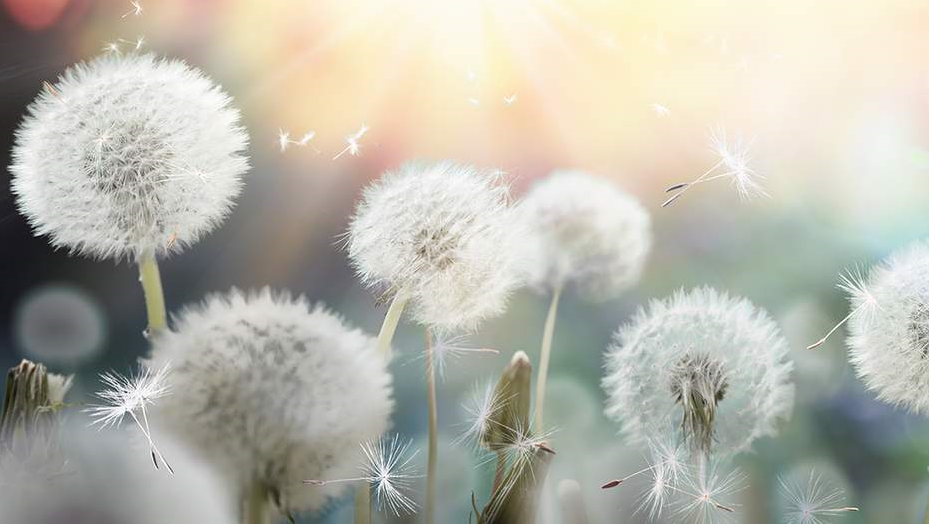
Conceptually and practically, Open Science and the FAIR principles are distinct but may have similarities. Therefore, data that can be considered 'open' and/or 'FAIR' need to be first discussed individually.
In this module, you will:- learn the basics of FAIR and Open, the research lifecycle and possible costs associated to these
- understand their relationship to each other
- learn about exceptions to Open Data and common arguments against being open, and the benefits of being open.
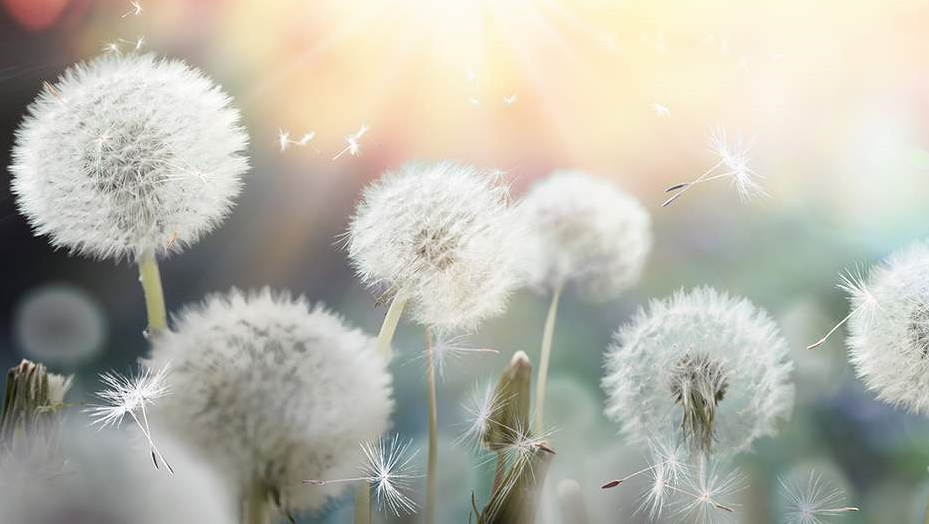
This course helps you to find the best open licence for your research outputs.
Licensing your research outputs is an important part of practicing Open Science. In this course, you will:
- know what licences are, how they work, and how to apply them.
- understand how different types of licenses can affect research output reuse.
- know how to select the appropriate license for your research.
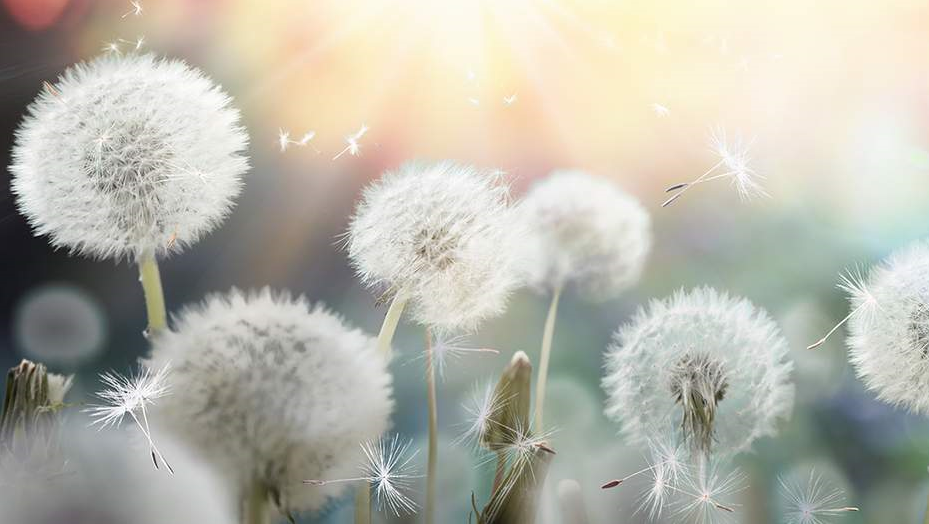
- introduce you to the key terms and explain what they mean in a practical sense.
- tell you how data management planning can help to make data FAIR from the very start of research projects.
- show you how you can use freely available tools to help assess the FAIRness of data.
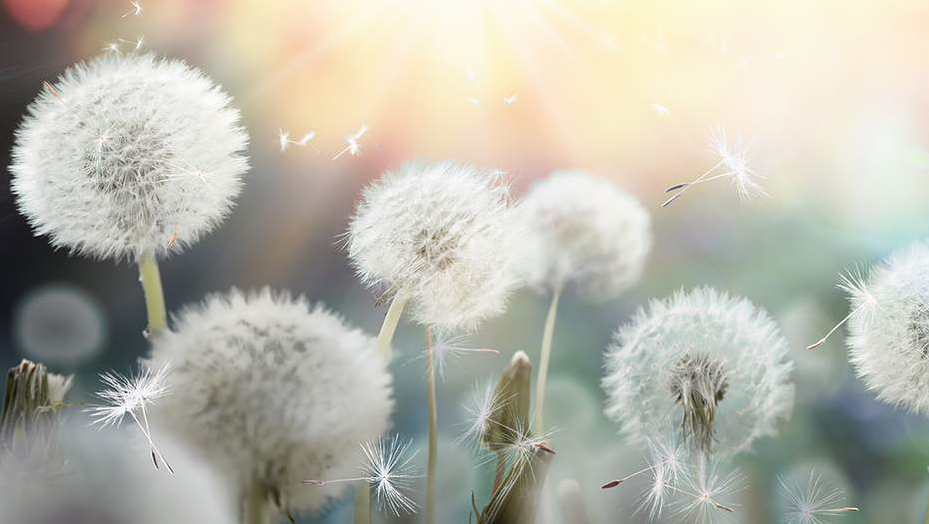
- understand how to publish your work openly and be aware of the advantages
- be able to find an OA publisher for your research
- know how to find a suitable repository to provide OA and archive your work
- know how to publish OA monographs
- understand funders' expectations and policies on OA
- be able to secure funding for Article Processing Charges (APCs) where applicable
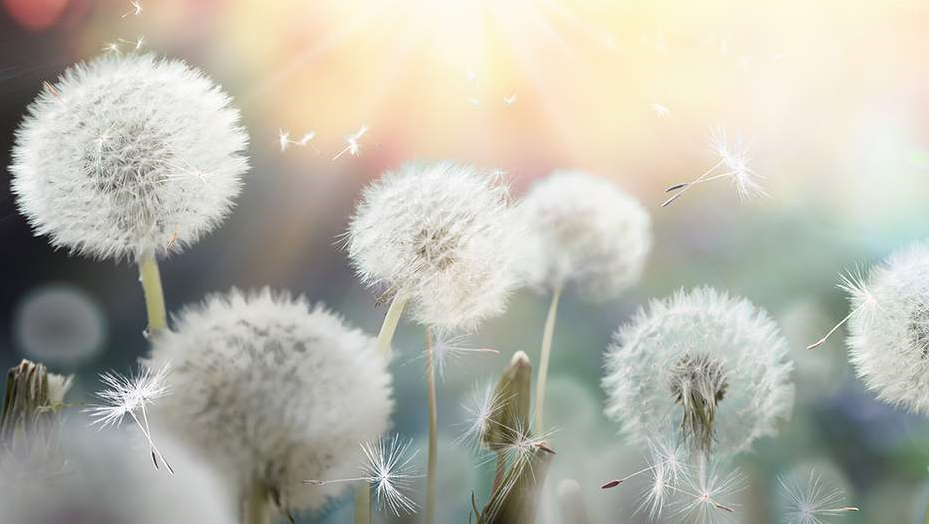
This course introduces Open Source Software (OSS) and workflow management as an emerging but critical component of Open Science.
The course explains the role of software sharing and sustainability in reproducibility, trust and longevity, and provides different perspectives around the sharing and reuse of computational code and methods, namely the software producer, the software reuser, and the non-coder with an interest either in reproducing research findings or in following experimental processes. You'll learn about useful resources and tools for sharing and exposing your code and workflows. Upon completing this course, you will:- understand the roles that open source software and open workflows play in supporting Open Science
- know how Open Science can support reproducibility
- be aware of issues to consider at different stages of the research lifecycle
- know about useful tools and resources to help you get started with using OSS and open workflows
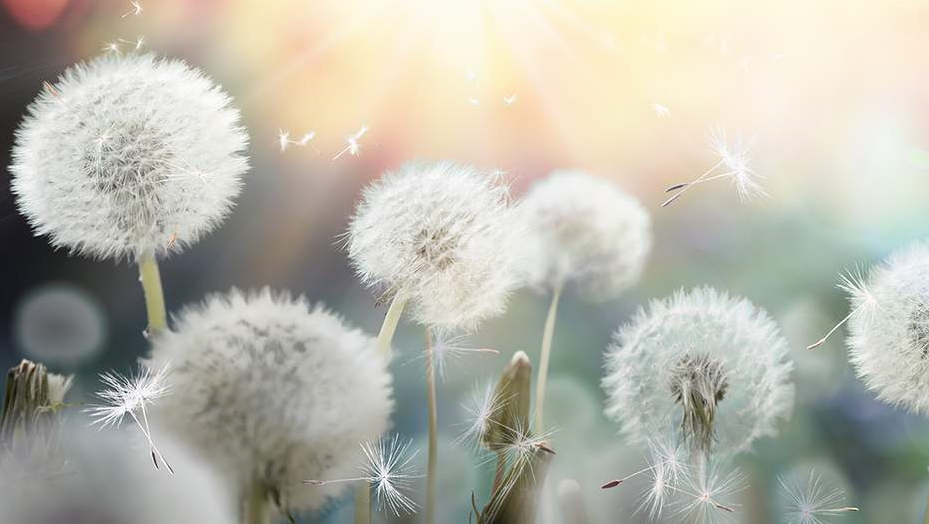
This course introduces some practical steps for opening up your research practices and how to meet expectations relating to openness from funders, publishers and peers.
We begin by exploring the practical implications of Open Science, and the benefits it can deliver for research integrity and public trust, as well as benefits you will accrue in your own work. (Please note that in some countries/regions, 'Open Science' is synonymous to 'Open Research' and these terms can be used interchangeably!) After a short elaboration of some useful rules of thumb, we move quickly onto some more practical steps towards meeting contemporary best practice in Open Science, and introduce some useful discipline specific resources. Upon completing this course, you will:
- be able to contextualise your research environment.
- be aware of the questions you should bear in mind as you progress through the key stages of your research.
- know about some discipline-specific resources to help you embed Open Science into work research practices.
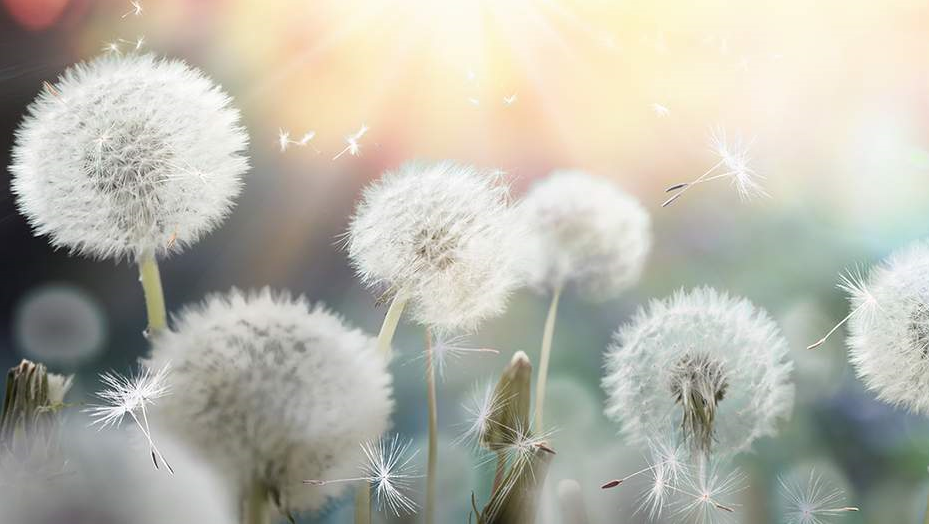
- introduce you to the key terms and explain what they mean in a practical sense.
- tell you how data management planning can help to make data FAIR from the very start of research projects.
- show you how you can use freely available tools to help assess the FAIRness of data.